In a recent interview, Stuart Tait, CTO of Tax and Legal at KPMG UK, compared the introduction of generative AI solutions in the financial sector to “the shift from typewriters to word processors”. This striking analogy perfectly illustrates the transformative potential of these technologies. Generative AI is already delivering tangible value across a wide range of financial tasks, and it looks set to become even more important in the future as its adoption continues to grow in this important sector.
Generative AI is generating considerable excitement. Many believe these tools will dramatically improve operational efficiency in the financial sector, enhance customer experiences, and streamline regulatory compliance. While this is certainly possible, there are still potential hurdles that could hinder progress. One of the most critical challenges is maintaining a steady supply of clean, relevant, and accurate data, especially as businesses increasingly rely on AI to make decisions.
DATA IS THE LIFEBOY OF AI
It must be acknowledged that despite all the enthusiasm, the crucial role of data in the reliability of AI systems has been somewhat overlooked. AI is often portrayed as a quasi-magical, sentient technology that operates autonomously, without intervention or assistance. However, this depiction is far from the truth. At the heart of every AI system is data, aptly described as the “engine” of these technologies. Therefore, if data is imprecise, incorrect, or irrelevant, the AI systems built on it will inevitably inherit these flaws.
In the financial sector, this crucial consideration cannot be ignored. Companies that want to develop AI systems must ensure that they do so with the high-quality data that these systems require. Furthermore, to fully exploit the potential of AI in the financial sector, companies need systems that provide easy access to relevant data, ensuring that data is properly formatted for incorporation into AI systems. Without these systems in place, AI systems will struggle to deliver the long-term transformative impact that many observers already seem to believe is inevitable.
PROVIDE HIGH VALUE INFORMATION
In this regard, I agree with Rohit Sehgal, Founder and CEO of Vincilium, who recently said that “AI needs data more than data needs AI.” The real value of AI lies in the improved analysis and insights derived from high-quality data. Ultimately, if the data fed to these systems is insufficient, difficult to use, interpret or access, then poor results are to be expected. In finance, this renders the systems unusable and exacerbates the very problems they were designed to solve.
In an era of strict regulation and increased compliance requirements, such an outcome could be particularly damaging. Inaccurate forecasts, biased outcomes, and flawed decision-making could put financial institutions at risk, damage customer relationships, and result in costly fines. To avoid this, companies must ensure that AI systems are trained exclusively on high-quality, diverse, and comprehensive datasets. Unfortunately, sourcing this data in today’s complex environment can be a challenge.
DATA SOURCING CHALLENGES
Financial institutions often face significant barriers to accessing valuable data due to outdated systems. These outdated platforms, which still store large amounts of critical information in a fragmented and siloed form, can be difficult to integrate with modern data and AI systems, posing a barrier to effective data use. Additionally, data silos pose a major challenge because they fragment information across different departments or systems, leading to inconsistent or incomplete data sets. This fragmentation can significantly hamper AI development efforts.
Additionally, the struggle to obtain clean data is an ongoing problem. Financial data is often messy, unstructured, or outdated, requiring extensive cleaning, organization, and structuring before it can be used effectively. This process is time-consuming and complex, but essential to ensuring that AI systems can provide accurate and valuable insights, rather than being compromised by poor data quality. Fortunately, data management systems that can access data streams regardless of format, system, or silo can help alleviate this problem.
FINDING THE RIGHT APPROACH
To create AI solutions that financial institutions can truly trust, the first requirement is to have reliable and trustworthy data in an easily accessible format. This step is essential in the development process and deserves greater recognition from companies dedicated to this goal. The ability to consolidate and aggregate large volumes of data is crucial in this context, as it allows AI systems to discover, analyze and extract actionable insights effectively and efficiently.
With these solutions, financial institutions can confidently accelerate their AI adoption plans and begin to realize the transformative benefits many anticipate from the technology. By implementing robust data management techniques, businesses can ensure the delivery of accurate and up-to-date data, which is essential for systems to automate routine tasks previously performed by humans. This not only saves countless hours, but also enables more accurate forecasting and improved decision-making.
About the author
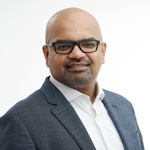
Karthik Jagannathan is the Head of Payments Consulting at Intixa global leader in transaction data management. With a proven track record of leading business and technology teams at leading banks and technology companies, Karthik specializes in building innovative payment solutions. His deep payments expertise, combined with extensive experience managing multinational projects, enables Intix to continue to leverage industry-leading data management techniques, providing businesses with clear, comprehensive and actionable insights at their fingertips. With over 20 years of experience in the financial services industry, Karthik is a recognized industry expert in instant payments and open banking. He has deep knowledge of compliance, data processing and how organizations can leverage technology innovation to drive meaningful changes in payments that directly benefit end users. At Intix, he is focused on helping clients navigate global regulatory changes in the age of increased compliance.
Subscribe to insideAI news for free newsletter.
Join us on Twitter: https://twitter.com/InsideBigData1
Join us on LinkedIn: https://www.linkedin.com/company/insideainews/
Join us on Facebook: https://www.facebook.com/insideAINEWSNOW